Smart Materials in Medical Applications: An Overview of Machine Learning-Based Futures
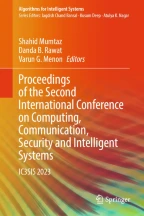
Smart materials (SMs) are a revolutionary advancing technology that can improve patient care in medical applications. Medical systems will be more efficient and effective in the future thanks to machine learning (ML) algorithms. Hence, in this paper, we present a look at ML-based medical futures as the foundation of our study of SMs. To realize the potential of SMs and ensure they are used responsibly, we identify areas of study that require consideration. Thus, this paper discusses the fabrication of SMs in particular hydrogels and their potential applications in biomedicine. SMs and hydrogels are explored in detail in this review, including techniques used to make hydrogels smart/intelligent by eliciting stimuli-responsiveness and environmental cue sensitivity. Moreover, the process of crosslinking polymers with reactive components and diverse substances is explained. We also discuss how intelligent hydrogels can be applied to wound healing, drug delivery, and tissue engineering, alongside stimuli-responsive hydrogels. Applied to medical and machine learning applications, this review opens the door to smart materials.
This is a preview of subscription content, log in via an institution to check access.
Access this chapter
Subscribe and save
Springer+ Basic
€32.70 /Month
- Get 10 units per month
- Download Article/Chapter or eBook
- 1 Unit = 1 Article or 1 Chapter
- Cancel anytime
Buy Now
Price includes VAT (France)
eBook EUR 192.59 Price includes VAT (France)
Hardcover Book EUR 242.64 Price includes VAT (France)
Tax calculation will be finalised at checkout
Purchases are for personal use only
References
- Chakrapani G, Zare M, Ramakrishna S (2022) Intelligent hydrogels and their biomedical applications. Mater Adv Google Scholar
- Sagdic K, Eş I, Sitti M, Inci F (2022) Smart materials: rational design in biosystems via artificial intelligence. Trends Biotechnol Google Scholar
- Wang L, Zhou M, Xu T, Zhang X (2022) Multifunctional hydrogel as wound dressing for intelligent wound monitoring. Chem Eng J 433:134625 ArticleGoogle Scholar
- Owh C, Ho D, Loh XJ, Xue K (2022) Towards machine learning for hydrogel drug delivery systems. Trends Biotechnol Google Scholar
- Holzinger A, Keiblinger K, Holub P, Zatloukal K, Müller H (2023) AI for life: trends in artificial intelligence for biotechnology. New Biotechnol 74:16–24 ArticleGoogle Scholar
- Bharatiya D, Patra S, Parhi B, Swain SK (2023) A materials science approach towards bioinspired polymeric nanocomposites: a comprehensive review. Int J Polym Mater Polym Biomater 72(2):119–134 ArticleGoogle Scholar
- Bogue R (2014) Smart materials: a review of capabilities and applications. Assem Autom 34(1):16–22 ArticleGoogle Scholar
- Raji M, Halloub A, el Kacem Qaiss A, Bouhfid R (2023) Bioplastic‐based nanocomposites for smart materials. In: Handbook of bioplastics and biocomposites engineering applications. pp 457–470 Google Scholar
- Rossiter J, Hauser H (2016) Soft robotics—the next industrial revolution. IEEE Robot Autom Mag 23(3):17–20 ArticleGoogle Scholar
- Spaggiari A, Castagnetti D, Golinelli N, Dragoni E, Scirè Mammano G (2019) Smart materials: Properties, design and mechatronic applications. Proc Inst Mech Eng, Part L: J Mater: Des Appl 233(4):734–762 Google Scholar
- Amukarimi S, Ramakrishna S, Mozafari M (2021) Smart biomaterials—a proposed definition and overview of the field. Curr Opin Biomed Eng 19:100311 ArticleGoogle Scholar
- Jingcheng L, Reddy VS, Jayathilaka WA, Chinnappan A, Ramakrishna S, Ghosh R (2021) Intelligent polymers, fibers and applications. Polymers 13(9):1427 ArticleGoogle Scholar
- McCarthy J, Minsky ML, Rochester N, Shannon CE (2006) A proposal for the dartmouth summer research project on artificial intelligence, august 31, 1955. AI Mag 27(4):12–12 Google Scholar
- Dopico M, Gómez A, De la Fuente D, García N, Rosillo R, Puche J (2016) A vision of industry 4.0 from an artificial intelligence point of view. In: Proceedings on the international conference on artificial intelligence (ICAI). The Steering Committee of The World Congress in Computer Science, Computer Engineering and Applied Computing (WorldComp), p 407 Google Scholar
- Butler KT, Davies DW, Cartwright H, Isayev O, Walsh A (2018) Machine learning for molecular and materials science. Nature 559(7715):547–555 ArticleGoogle Scholar
- Li BH, Hou BC, Yu WT, Lu XB, Yang CW (2017) Applications of artificial intelligence in intelligent manufacturing: a review. Front Inf Technol Electron Eng 18:86–96 ArticleGoogle Scholar
- Ezell SJ (2016) A policy maker’s guide to smart manufacturing Google Scholar
- Pan Y (2016) Heading toward artificial intelligence 2.0. Engineering 2(4):409–413 Google Scholar
- Huang SH, Zhang HC (1994) Artificial neural networks in manufacturing: concepts, applications, and perspectives. IEEE Trans Compon Packag Manuf Technol Part A 17(2):212–228 ArticleMathSciNetGoogle Scholar
- Akceoglu GA, Saylan Y, Inci F (2021) A snapshot of microfluidics in point-of-care diagnostics: multifaceted integrity with materials and sensors. Adv Mater Technol 6(7):2100049 ArticleGoogle Scholar
- Hong W, Almomani A, Chen Y, Jamshidi R, Montazami R (2017) Soft ionic electroactive polymer actuators with tunable non-linear angular deformation. Materials 10(6):664 ArticleGoogle Scholar
- Zhang Z, Demir KG, Gu GX (2019) Developments in 4D-printing: a review on current smart materials, technologies, and applications. Int J Smart Nano Mater 10(3):205–224 ArticleGoogle Scholar
- Jin H, Abu-Raya YS, Haick H (2017) Advanced materials for health monitoring with skin-based wearable devices. Adv Healthcare Mater 6(11):1700024 ArticleGoogle Scholar
- Yun J, Jayababu N, Kim D (2020) Self-powered transparent and flexible touchpad based on triboelectricity towards artificial intelligence. Nano Energy 78:105325 ArticleGoogle Scholar
- Rocher P, El Medawar L, Hornez JC, Traisnel M, Breme J, Hildebrand HF (2004) Biocorrosion and cytocompatibility assessment of NiTi shape memory alloys. Scripta Mater 50(2):255–260 ArticleGoogle Scholar
- Nazarahari A, Canadinc D (2021) Prediction of the NiTi shape memory alloy composition with the best corrosion resistance for dental applications utilizing artificial intelligence. Mater Chem Phys 258:123974 ArticleGoogle Scholar
- Mehrpouya M, Gisario A, Rahimzadeh A, Nematollahi M, Baghbaderani KS, Elahinia M (2019) A prediction model for finding the optimal laser parameters in additive manufacturing of NiTi shape memory alloy. Int J Adv Manuf Technol 105:4691–4699 ArticleGoogle Scholar
- Mehrpouya M, Gisario A, Nematollahi M, Rahimzadeh A, Baghbaderani KS, Elahinia M (2021) The prediction model for additively manufacturing of NiTiHf high-temperature shape memory alloy. Mater Today Commun 26:102022 ArticleGoogle Scholar
- Velmurugan C, Senthilkumar V, Dinesh S (2018) Artificial neural network prediction of wire electrical discharge machining properties on sintered porous NiTi shape memory alloy. Mater Today: Proc 5(2):8382–8390 Google Scholar
- Saleh TA, Fadillah G, Ciptawati E (2021) Smart advanced responsive materials, synthesis methods and classifications: from Lab to applications. J Polym Res 28(6):197 ArticleGoogle Scholar
- de La Torre LG, Sipoli CC, Oliveira AF, Eş I, Pessoa AC, Vitor MT, et al (2017) Biopolymers for gene delivery applications. In: Biopolymer-based composites. Woodhead Publishing, pp 289–323 Google Scholar
- Carrico JD, Hermans T, Kim KJ, Leang KK (2019) 3D-printing and machine learning control of soft ionic polymer-metal composite actuators. Sci Rep 9(1):1–17 ArticleGoogle Scholar
- Lee YW, Ceylan H, Yasa IC, Kilic U, Sitti M (2020) 3D-printed multi-stimuli-responsive mobile micromachines. ACS Appl Mater Interfaces 13(11):12759–12766 ArticleGoogle Scholar
- Hoffman AS (2013) Stimuli-responsive polymers: Biomedical applications and challenges for clinical translation. Adv Drug Deliv Rev 65(1):10–16 ArticleGoogle Scholar
- Boztepe C, Künkül A, Yüceer M (2020) Application of artificial intelligence in modeling of the doxorubicin release behavior of pH and temperature responsive poly (NIPAAm-co-AAc)-PEG IPN hydrogel. J Drug Deliv Sci Technol 57:101603 ArticleGoogle Scholar
- Li F, Han J, Cao T, Lam W, Fan B, Tang W et al (2019) Design of self-assembly dipeptide hydrogels and machine learning via their chemical features. Proc Natl Acad Sci 116(23):11259–11264 ArticleGoogle Scholar
- Hesami M, Naderi R, Tohidfar M, Yoosefzadeh-Najafabadi M (2020) Development of support vector machine-based model and comparative analysis with artificial neural network for modeling the plant tissue culture procedures: effect of plant growth regulators on somatic embryogenesis of chrysanthemum, as a case study. Plant Methods 16:1–15 ArticleGoogle Scholar
- Boztepe C, Yüceer M, Künkül A, Şölener M, Kabasakal OS (2020) Prediction of the deswelling behaviors of pH-and temperature-responsive poly (NIPAAm-co-AAc) IPN hydrogel by artificial intelligence techniques. Res Chem Intermed 46:409–428 ArticleGoogle Scholar
- Gonzalez-Navarro FF, Stilianova-Stoytcheva M, Renteria-Gutierrez L, Belanche-Muñoz LA, Flores-Rios BL, Ibarra-Esquer JE (2016) Glucose oxidase biosensor modeling and predictors optimization by machine learning methods. Sensors 16(11):1483 ArticleGoogle Scholar
- Saylan Y, Erdem Ö, Inci F, Denizli A (2020) Advances in biomimetic systems for molecular recognition and biosensing. Biomimetics 5(2):20 ArticleGoogle Scholar
- Inan H, Wang S, Inci F, Baday M, Zangar R, Kesiraju S et al (2017). Isolation, detection, and quantification of cancer biomarkers in HPV-associated malignancies. Sci Rep 7(1):1–11 Google Scholar
- Martynko E, Kirsanov D (2020) Application of chemometrics in biosensing: a brief review. Biosensors 10(8):100 ArticleGoogle Scholar
- Un KC, Wong CK, Lau YM, Lee JCY, Tam FCC, Lai WH, et al (2021) Observational study on wearable biosensors and machine learning-based remote monitoring of COVID-19 patients. Sci Rep 11(1):4388 Google Scholar
- Bousselham A, Bouattane O, Youssfi M, Raihani A (2019) Towards reinforced brain tumor segmentation on MRI images based on temperature changes on pathologic area. Int J Biomed Imaging Google Scholar
- Zhao Y, Gafita A, Vollnberg B, Tetteh G, Haupt F, Afshar-Oromieh A, et al (2020) Deep neural network for automatic characterization of lesions on 68 Ga-PSMA-11 PET/CT. Eur J Nucl Med Mol Imaging 47:603–613 Google Scholar
- Park C, Na KJ, Choi H, Ock CY, Ha S, Kim M, et al (2020) Tumor immune profiles noninvasively estimated by FDG PET with deep learning correlate with immunotherapy response in lung adenocarcinoma. Theranostics 10(23):10838 Google Scholar
- Alafeef M, Srivastava I, Pan D (2020) Machine learning for precision breast cancer diagnosis and prediction of the nanoparticle cellular internalization. ACS Sensors 5(6):1689–1698 ArticleGoogle Scholar
- Li H, Xu C, Xin B, Zheng C, Zhao Y, Hao K et al (2019) 18F-FDG PET/CT radiomic analysis with machine learning for identifying bone marrow involvement in the patients with suspected relapsed acute leukemia. Theranostics 9(16):4730 ArticleGoogle Scholar
- Rezaei A, Ejupi A, Gholami M, Ferrone A, Menon C (2018) Preliminary investigation of textile-based strain sensors for the detection of human gait phases using machine learning. In: 2018 7th IEEE international conference on biomedical robotics and biomechatronics (Biorob). pp 563–568 Google Scholar
- Sundaram S, Kellnhofer P, Li Y, Zhu JY, Torralba A, Matusik W (2019) Learning the signatures of the human grasp using a scalable tactile glove. Nature 569(7758):698–702 ArticleGoogle Scholar
- Liu K, Tebyetekerwa M, Ji D, Ramakrishna S (2020) Intelligent materials. Matter 3(3):590–593 ArticleGoogle Scholar
- Tian S, Yang W, Le Grange JM, Wang P, Huang W, Ye Z (2019) Smart healthcare: making medical care more intelligent. Global Health J 3(3):62–65 ArticleGoogle Scholar
- Pishavar E, Khosravi F, Naserifar M, Rezvani Ghomi E, Luo H, Zavan B et al (2021) Multifunctional and self-healable intelligent hydrogels for cancer drug delivery and promoting tissue regeneration in vivo. Polymers 13(16):2680 ArticleGoogle Scholar
- Joyce K, Fabra GT, Bozkurt Y, Pandit A (2021) Bioactive potential of natural biomaterials: identification, retention and assessment of biological properties. Signal Transduct Target Ther 6(1):122 ArticleGoogle Scholar
- Rezvani Ghomi E, Nourbakhsh N, Akbari Kenari M, Zare M, Ramakrishna S (2021) Collagen-based biomaterials for biomedical applications. J Biomed Mater Res B Appl Biomater 109(12):1986–1999 ArticleGoogle Scholar
- Papathanasiou KE, Turhanen P, Brückner SI, Brunner E, Demadis KD (2017) Smart, programmable and responsive injectable hydrogels for controlled release of cargo osteoporosis drugs. Sci Rep 7(1):1–9 ArticleGoogle Scholar
- Han K, Nam J, Xu J, Sun X, Huang X, Animasahun O et al (2021) Generation of systemic antitumour immunity via the in situ modulation of the gut microbiome by an orally administered inulin gel. Nat Biomed Eng 5(11):1377–1388 ArticleGoogle Scholar
- Mantha S, Pillai S, Khayambashi P, Upadhyay A, Zhang Y, Tao O, et al (2019) Smart hydrogels in tissue engineering and regenerative medicine. Materials 12(20):3323 Google Scholar
- Montero FE, Rezende RA, Da Silva JV, Sabino MA (2019) Development of a smart bioink for bioprinting applications. Front Mech Eng 5:56 ArticleGoogle Scholar
- Cui R, Wu Q, Wang J, Zheng X, Ou R, Xu Y et al (2021) Hydrogel-by-design: smart delivery system for cancer immunotherapy. Front Bioeng Biotechnol 9:723490 ArticleGoogle Scholar
- Schwall CT, Banerjee IA (2009) Micro-and nanoscale hydrogel systems for drug delivery and tissue engineering. Materials 2(2):577–612 ArticleGoogle Scholar
- Wang W, Narain R, Zeng H (2020) Hydrogels. In: Polymer science and nanotechnology. Elsevier, pp 203–244 Google Scholar
- Grenier J, Duval H, Barou F, Lv P, David B, Letourneur D (2019) Mechanisms of pore formation in hydrogel scaffolds textured by freeze-drying. Acta Biomater 94:195–203 ArticleGoogle Scholar
- Zhang Y, Huang Y (2021) Rational design of smart hydrogels for biomedical applications. Front Chem 8:615665 ArticleGoogle Scholar
- Pati F, Jang J, Ha DH, Won Kim S, Rhie JW, Shim JH et al (2014) Printing three-dimensional tissue analogues with decellularized extracellular matrix bioink. Nat Commun 5(1):3935 ArticleGoogle Scholar
- Peppas NA, Hilt JZ, Khademhosseini A, Langer R (2006) Hydrogels in biology and medicine: from molecular principles to bionanotechnology. Adv Mater 18(11):1345–1360 ArticleGoogle Scholar
- Caldorera-Moore M, Peppas NA (2009) Micro-and nanotechnologies for intelligent and responsive biomaterial-based medical systems. Adv Drug Deliv Rev 61(15):1391–1401 ArticleGoogle Scholar
- Liang S, Qiu X, Yuan J, Huang W, Du X, Zhang L (2018) Multiresponsive kinematics and robotics of surface-patterned polymer film. ACS Appl Mater Interfaces 10(22):19123–19132 ArticleGoogle Scholar
- Tabor J, Chatterjee K, Ghosh TK (2020) Smart textiles: smart textile‐based personal thermal comfort systems: current status and potential solutions (Adv Mater Technol 5/2020). Adv Mater Technol 5(5):2070025 Google Scholar
- Reker D, Rybakova Y, Kirtane AR, Cao R, Yang JW, Navamajiti N et al (2021) Computationally guided high-throughput design of self-assembling drug nanoparticles. Nat Nanotechnol 16(6):725–733 ArticleGoogle Scholar
- Suwardi A, Wang F, Xue K, Han MY, Teo P, Wang P et al (2022) Machine learning-driven biomaterials evolution. Adv Mater 34(1):2102703 ArticleGoogle Scholar
- Castro BM, Elbadawi M, Ong JJ, Pollard T, Song Z, Gaisford S et al (2021) Machine learning predicts 3D printing performance of over 900 drug delivery systems. J Control Release 337:530–545 ArticleGoogle Scholar
- Li F, Han J, Cao T, Lam W, Fan B, Tang W et al (2019) Design of self-assembly dipeptide hydrogels and machine learning via their chemical features. Proc Natl Acad Sci 116(23):11259–11264 Google Scholar
- Parikh BH, Liu Z, Blakeley P, Lin Q, Singh M, Ong JY et al (2022) A bio-functional polymer that prevents retinal scarring through modulation of NRF2 signalling pathway. Nat Commun 13(1):2796 ArticleGoogle Scholar
- Garcia-del Rio L, Diaz-Rodriguez P, Landin M (2020) New tools to design smart thermosensitive hydrogels for protein rectal delivery in IBD. Mater Sci Eng, C 106:110252 ArticleGoogle Scholar
- Xu F, Corbett B, Bell S, Zhang C, Budi Hartono M, Farsangi ZJ et al (2019) High-throughput synthesis, analysis, and optimization of injectable hydrogels for protein delivery. Biomacromol 21(1):214–229 Google Scholar
- Bannigan P, Bao Z, Hickman RJ, Aldeghi M, Häse F, Aspuru-Guzik A, Allen C (2023) Machine learning models to accelerate the design of polymeric long-acting injectables. Nat Commun 14(1):35 ArticleGoogle Scholar
- Vigata M, Meinert C, Hutmacher DW, Bock N (2020) Hydrogels as drug delivery systems: a review of current characterization and evaluation techniques. Pharmaceutics 12(12):1188 ArticleGoogle Scholar
- Yamada H, Liu C, Wu S, Koyama Y, Ju S, Shiomi J, Morikawa J, Yoshida R (2019) Predicting materials properties with little data using shotgun transfer learning. ACS Cent Sci 5(10):1717–1730 ArticleGoogle Scholar
- Rashid MBMA, Toh TB, Hooi L, Silva A, Zhang Y, Tan PF, et al (2018) Optimizing drug combinations against multiple myeloma using a quadratic phenotypic optimization platform (QPOP). Sci Transl Med 10(453):eaan0941 Google Scholar
- Ho D (2020) Artificial intelligence in cancer therapy. Science 367(6481):982–983 ArticleGoogle Scholar
- Zhou X, Hu Y, Wu J, Liang W, Ma J, Jin Q (2022) Distribution bias aware collaborative generative adversarial network for imbalanced deep learning in industrial IoT. IEEE Trans Ind Inf 19(1):570–580 ArticleGoogle Scholar
- Zhou X, Liang W, Yan K, Li W, Kevin I, Wang K et al (2022) Edge-enabled two-stage scheduling based on deep reinforcement learning for internet of everything. IEEE Internet Things J 10(4):3295–3304 Google Scholar
- Zhou X, Liang W, Li W, Yan K, Shimizu S, Kevin I, Wang K (2021) Hierarchical adversarial attacks against graph-neural-network-based IoT network intrusion detection system. IEEE Internet Things J 9(12):9310–9319 ArticleGoogle Scholar
- Wang F, Wang L, Li G, Wang Y, Lv C, Qi L (2021) Edge-cloud-enabled matrix factorization for diversified APIs recommendation in mashup creation. World Wide Web 1–21 Google Scholar
- Zhou X, Liang W, Kevin I, Wang K, Yang LT (2020) Deep correlation mining based on hierarchical hybrid networks for heterogeneous big data recommendations. IEEE Trans Comput Soc Syst 8(1):171–178 ArticleGoogle Scholar
- Qi L, Yang Y, Zhou X, Rafique W, Ma J (2021) Fast anomaly identification based on multiaspect data streams for intelligent intrusion detection toward secure industry 4.0. IEEE Trans Ind Inform 18(9):6503–6511 Google Scholar
- Wu S, Shen S, Xu X, Chen Y, Zhou X, Liu D et al (2022) Popularity-aware and diverse web APIs recommendation based on correlation graph. IEEE Trans Comput Soc Syst 10(2):771–782 ArticleGoogle Scholar
- Zhou X, Xu X, Liang W, Zeng Z, Yan Z (2021) Deep-learning-enhanced multitarget detection for end–edge–cloud surveillance in smart IoT. IEEE Internet Things J 8(16):12588–12596 ArticleGoogle Scholar
- Dai H, Yu J, Li M, Wang W, Liu AX, Ma J et al (2022) Bloom filter with noisy coding framework for multi-set membership testing. IEEE Trans Knowl Data Eng Google Scholar
- Kong L, Li G, Rafique W, Shen S, He Q, Khosravi MR, et al (2022) Time-aware missing healthcare data prediction based on ARIMA model. IEEE/ACM Trans Comput Biol Bioinform Google Scholar
Author information
Authors and Affiliations
- Department of Biomedical Engineering, Meybod University, Meybod, Iran Khosro Rezaee & Mojtaba Ansari
- Shandong Provincial University Laboratory for Protected Horticulture, Weifang University of Science and Technology, 262799, Weifang, Shandong, China Mohamadreza Khosravi
- Department of Medical Physics and Engineering, School of Medicine, Shiraz University of Medical Sciences, Shiraz, Iran Mohamadreza Khosravi
- Khosro Rezaee